One of Metail’s highlights from last year was Snap selecting us as their first Shopping and Fashion GHOST Fellow đȘđ» Whilst this was a big deal for our team you might be wondering âWhat is GHOST?â or âWhy was Metail so excited by this opportunity?â
GHOST is Snapâs AR Innovation Lab and was designed to support the next generation of augmented reality products that help people shop, learn, play, and solve everyday problems around the world.
Snap launched GHOST with six different tracks including areas which people might not have anticipated such as Education and Utility. Snap choosing to include a Utility track was definitely a positive signal for Metail. It gave us proof that they were thinking beyond social and instead how their augmented reality technology could be used to solve problems in peopleâs everyday lives. Furthermore, GHOST has a whole track dedicated to Shopping & Fashion - “What a perfect fit with Metail!” we naturally thought so we duly set about preparing our application.
Metail’s GHOST Fellowship Aims
The funding available in the GHOST Fellowship meant that we were able to structure our application around two core aims:
- Increasing the amount of clothes that can be tried on with AR
- Increasing the usefulness of apparel AR try-on
The first aim builds upon Metailâs work with Browzwear and our EcoShot product. If you are not familiar with apparel-specific 3D CAD software you may not have heard of Browzwear before. You will however be familiar with their customers which includes the likes of PUMA, The North Face, Tommy Hilfiger and Walmart. Browzwearâs software enables the creation of true-to-life virtual 3D versions of physical clothes which minimises the production of physical samples in the development process and speeds up time-to-market without sacrificing product quality. Metailâs EcoShot product allows Browzwear users to simulate and visualise clothes on professional models instead of avatars. It is used by the likes of PUMA, The North Face, Tommy Hilfiger and Walmart! By combining accurate physics based simulation and realistic ray tracing rendering, Metail allows Browzwear users to create true-to-life visualisations of their 3D garments on professional models.
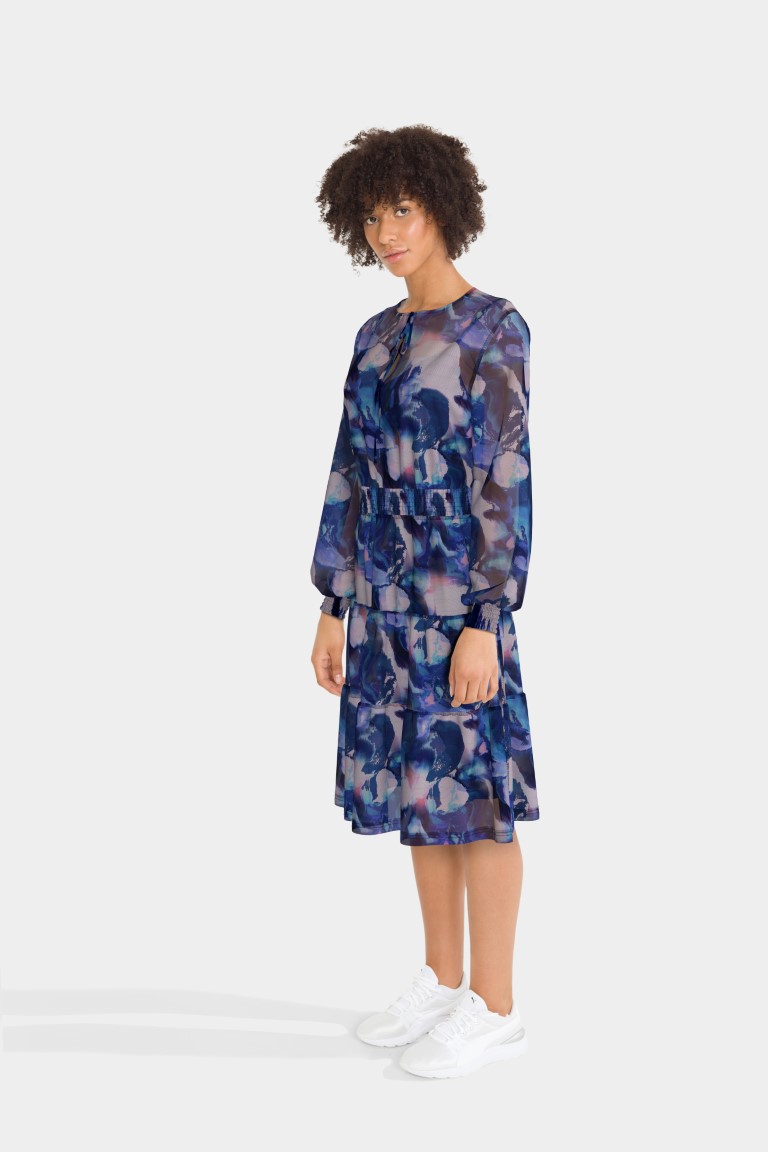
EcoShot and 3D CAD software like Browzwear are both geared towards providing accuracy in the apparel design and development process. They therefore require a lot of compute power and time to create these high quality, accurate results. Conversely, augmented reality is first and foremost geared towards interactivity and letting consumers see results in real-time on consumer devices such as mobile phones and smart glasses. As a result, the detailed and accurate 3D garments from software like Browzwear cannot be used directly within AR experiences. That is why our first GHOST aim is to devise a pipeline that lets Browzwear users quickly and easily make their existing virtual garments suitable for Lens Studio and AR try-on inside of Snapchat.
In a subsequent blog we will be sharing more details about this pipeline. The article below instead focuses on Metail’s second GHOST aim of increasing the usefulness of apparel AR try-on and how Snap are helping Metail to achieve this through the GHOST Fellowship.
Environment + Realism
A key aspect to making augmented reality appear more realistic is to make the digital assets look like they belong in the real world camera input feed. If the camera input shows a dark room then a brightly lit virtual garment would look out of place. Similarly a sharp, detailed virtual garment would look out of context if low light causes the camera input to be noisy rather than crisp. You can see an example of both in the image below:
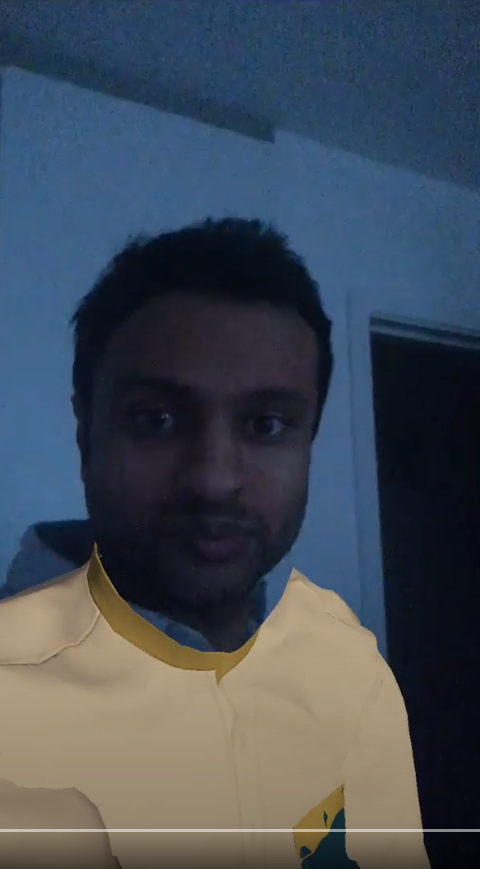
This is why the userâs environment is so important to making augmented reality look more realistic; it provides the key to making the virtual assets look grounded and in keeping with the userâs current location. Here is an AR example showing a virtual Apple HomePod mini next to its real physical world counterpart.
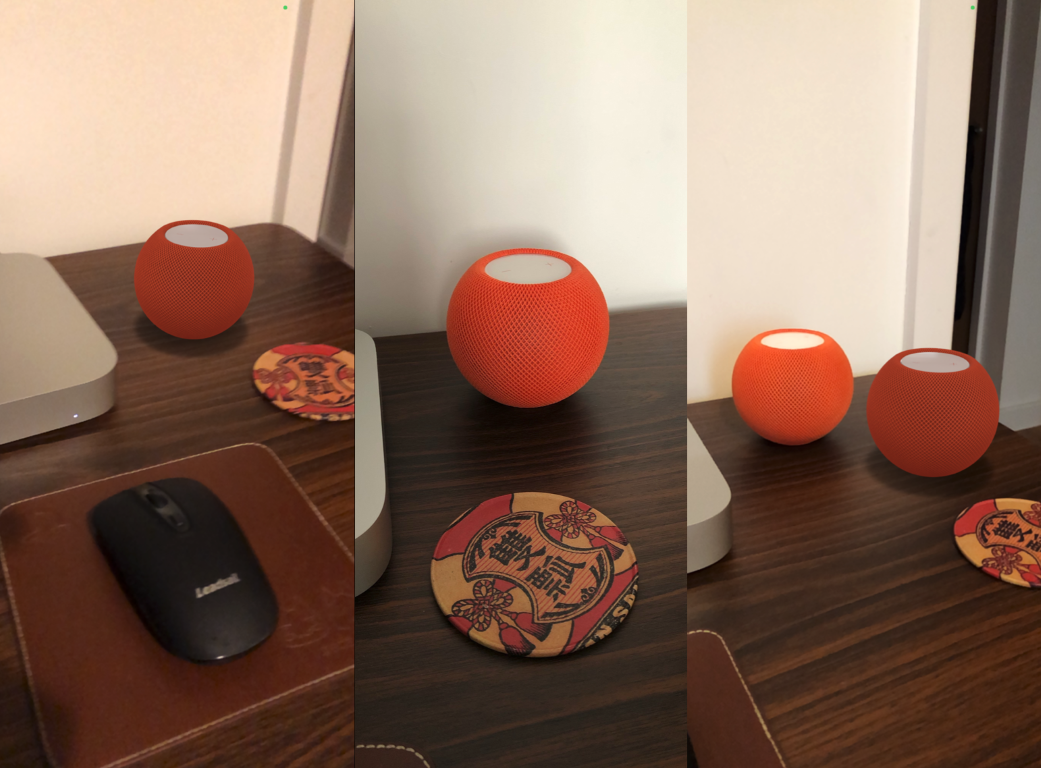
The challenge is that it is notoriously difficult to estimate the userâs environment and their current lighting from a single camera input feed. We believe the AR representation above is one of the more realistic examples but the slight difference in colour hues shows the effect the light estimation can have on the end result.
Our team at Metail has also learnt from first hand experience with the difficulty of estimating the lighting in a userâs scene after our team spent a lot of time exploring if Appleâs AR Kit could be used to generate a realistic HDR of the present light environment for use in 3D CAD software like Browzwear and also augmented reality. Our tests revealed a huge amount of variability in the environment capture of the same scene when using this approach.
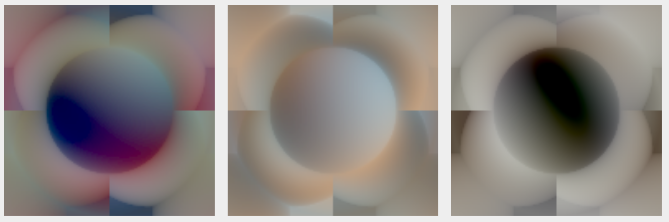
This large variability when using standard AR components provided by the likes of Apple meant that we had to pause the line of work. However, we knew it was still an important area which had to be tackled if we wanted to deliver more realistic AR try-on for clothes that are designed to be worn in the physical world. That’s why we immediately jumped at the chance when Snap gave us the opportunity to test their new machine learning based approaches to environment matching. Snap has now publicly announced these features at this year’s Snap Partner Summit alongside an AR try-on lens Metail created which means that we are finally able to share more information with you all!
Lens Studio’s New ML Environment Matching features
Snap’s approach to helping AR creators match their users’ environment involves using machine learning to automatically estimate the appropriate results for three elements:
- Light
- Blur
- Noise
The Snap team is working on making these estimates the default option for all AR lenses. In the meantime, AR creators will be able to enable these optional enhancements in lenses which they create.
The blur and noise estimations were the first new features we tested. These machine learning algorithms help to render 3D objects with realistic camera distortions. In this image below you can see how this estimation really helps to improve the realism of the augmented results as the top now looks more in keeping with the noisy and blurry camera input from the dark room.
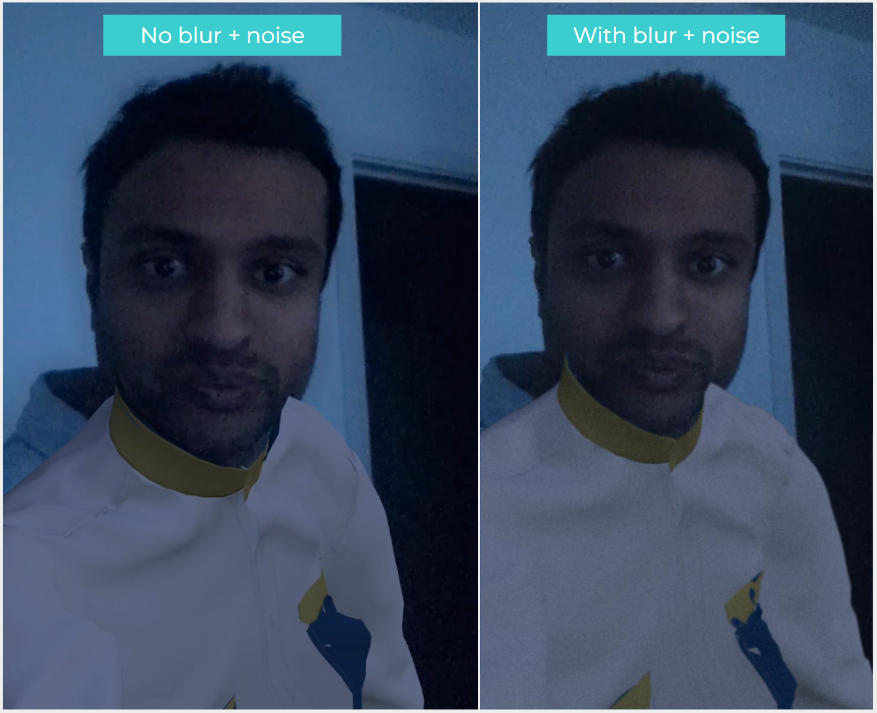
There were some edge cases which resulted in too much blur and noise (e.g., some portrait-mode photos with a sharp, clear face in front of a blurry, noisy background). In some well-light situations the additional noise can become a bit distracting. However, we ultimately felt that the features added to the realism in most examples we tested, especially in poorly lit environments; think night time and dark indoor scenes such as a nightclub. We believe the noise and blur estimation will make the AR try experience feel far more real in these scenes.
After our challenges and frustrations with Appleâs AR Kit environmental lighting information, the next feature we tested was the one we were most excited to try; Snapâs new ML light estimation.
This new machine learning model for Lens Studio predicts the lighting from a humanâs face. It can then be applied in a physically-based manner on 3D objects such as virtual garments.
One of the moments which made me truly appreciate the value of this new estimation was when I tested switching off the light of the room I was in. Seeing how quickly the garment updated and became dark to reflect the lower light in the room was a moment of surprise and delight!
My colleague Samson had a similar reaction when testing the new light estimation outside:
âI was surprised by how responsive it is. One example was when I was walking from direct sunlight into shade with trees. The ML environment matching was keeping up in real-time with the both sudden and also subtle changes of light. This level of material interaction with the real world environment gives a stronger sense of the 3D garment feeling real"
You can try it for yourself on the Snapchat app on your phone by either clicking this link or scanning the Snapcode below with the app:
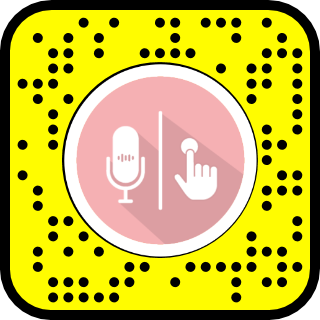
The ease of implementation was also a pleasant surprise to our computer graphics expert David as minimal material changes were required to make the new estimation work. After adding a couple of extra inputs the rest of the material works as expected. Impressing David is not an easy task as he’s an expert on both colour and computer graphics. When I saw the big đ reaction he gave on Slack after testing the new light estimation, I knew for certain Snap had provided another key feature to help us improve apparel AR try-on experiences.
Why do Metail think these features are a big deal?
The answer can be summed up in two words: realism and usefulness.
Let’s start with the former - realism. At Metail, we already firmly believe in the power of AR try-on as a tool that will help consumers to gain a faster and better understanding of whether a design is relevant and right for them. We also believe itâs a slightly different story for apparel brands at the moment. Our experience to date is that they still need a bit more convincing. Thatâs why making a brandâs clothes look more realistic in AR experiences has the power to move them away from feeling hesitant about AR try-on and instead excited about the prospect of letting people all around the world try on their clothes. When you think about how much time brands spend thinking about the aesthetics of their designs this immediately becomes understandable. Naturally they will want their digital representations to live up to these aesthetics and will be concerned if the quality of AR representations detract from their designs. This is part of the reason why realistic rendering of virtual garments in AR experiences is so important to a company like Metail.
The second word relates to our second GHOST Fellowship aim: Increasing the usefulness of apparel AR try-on. At Metail weâve broken this down into two main areas of investigation during our GHOST Fellowship:
- realistically convey the fit of clothing that is designed to be worn in the physical world
- realistically convey the look of clothing that is designed to be worn in the physical world
As part of the second area, Metail had already been leveraging the capabilities within Lens Studio to give consumers a better understanding of the different shades an outfit can take in different physical locations. Our try-on lenses give consumers the opportunity to see how the look of an outfit on them will change if they are outside on a sunny day compared to an overcast day. Or at home versus at the office.
However, we still believe that the usefulness of AR try-on lenses increases even more if consumers are able to see a realistic representation of how an outfit will look in their present location alongside these other environments. Whilst this was always technically possible, we had been hesitant about letting userâs see clothes in their current location due to the aforementioned difficulty in estimating the lighting in the userâs environment. If you get this light estimate wrong the AR clothes can look completely different to how youâd expect the physical clothes to look in the same scene and runs the risk of sparking an objection from the clothing brand. As a result, we had been wary about showing this type of representation to consumers and brands. Snapâs new ML environment matching features have changed our stance and have given us the confidence to include this useful âin-situâ visualisation in all of our future AR try-on lenses.
If you are involved in the apparel space and are excited by the prospect of AR letting people try on your clothes, do get in touch with us via the contact form on our Augmented Reality page.